Did AI Deny People from Getting Credits and Forbrukslån
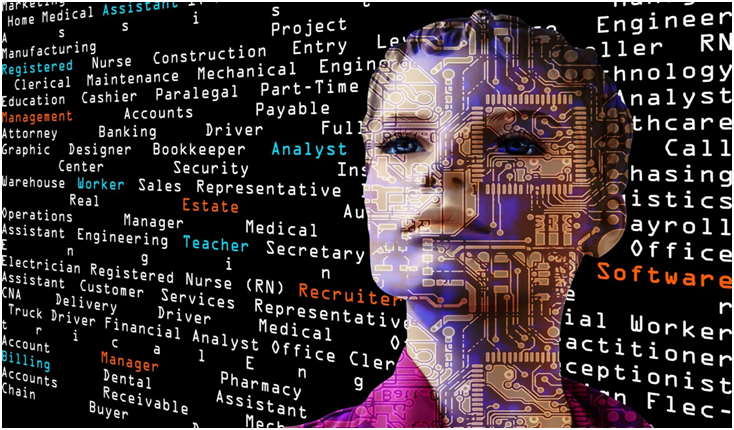
Individuals who apply for loans from financial institutions like conventional banks, credit card firms, or lending companies, and are turned down, are owed a considerable explanation of why they were turned down. It is an excellent idea – since it can help teach individuals how to repair their blemished credits – and it is the law (the ECOA or Equal Credit Opportunity Act). Getting answers was not a massive issue in past years when people made these decisions.
But in today’s world, like artificial intelligence or AI systems increasingly helping or replacing individuals making loan decisions, getting these explanations has become a lot more challenging. Usually, loan officers who reacted to applications could tell borrowers there were issues with their income levels, work history, or whatever the problem was.
How AI started? Click this site to find out more.
But computerized or automated systems that use complicated machine learning models are hard to explain, even for people who are in this business for many years. Consumer loan decisions are one way this issue arises. Similar issues exist in online marketing, criminal justice, and health care.
Our interest in this subject matter started when research groups discovered gender bias in how Internet advertisements were targeted but couldn’t explain why these things happened. All the industries mentioned above, and many others, that use these machine learning platforms to help them analyze processes and make the right decisions have one year to get much better at explaining how their financial systems work.
In 2018, the General Data Protection Regulation took effect in countries under the European Union, including a section giving individuals the right to get explanations for automated decisions that affect their financial situations. What shape should these explanations from experts take, and can organizations actually provide them?
Identifying important reasons
One way to tell why automated decisions came out the way they did is to find out the various factors that were pretty influential in the decision-making. How many credit denial decisions were made because the borrower did not make enough money or because they had failed to repay previous loans or credits?
Some experts created ways to measure the relative influences of every factor. They called it the quantitative input influence. In addition to providing a better understanding of individual decisions, the measurement can also provide a better understanding of group decisions: Did algorithms are denied credit because of financial issues, like how much applicants already owes other credit firms or financial institution? Or was the borrower’s ZIP code an essential factor – suggesting basic demographics like the race of borrowers might have a considerable influence on the decision-making?
Check out sites like https://www.sammenlignforbrukslån.net/ to find out more about this subject.
Capturing causation
When systems make conclusions based on various factors, it is imperative to identify which factors can influence decisions, as well as their relative contributions. For instance, imagine credit-decision systems that take just two inputs, a borrower’s DTI or debt-to-income ratio and their race, as well as loans that have only been shown to Caucasians.
Knowing how much every factor contributed to decisions can help organizations understand whether it is a legit system or whether their decision is discriminating. Explanations could just take a closer look at the inputs and outcomes and observe similarities or correlations – non-Caucasian individuals did not get credit.
But these explanations are too simplistic. Suppose non-Caucasian individuals who were denied credits also had lower incomes compared to Caucasians whose applications were pretty successful. Then these explanations can’t tell organizations whether the borrower’s DTI or race caused such denials.
These methods can provide these types of details. Telling differences mean organizations can check out whether their systems are unjustly discriminating or looking at legit criteria such as applicants’ finances. To measure the influence of factors like race in a certain credit decision, organizations redo their application process, keeping the DTI ratio the same but tweaking applicants’ race.
If changing the race has a certain effect on the loan outcome, they will know that it is a deciding factor. If not, they can conclude that the algorithm they are using is looking only at the financial details of applicants. In addition to recognizing factors that can cause these situations, companies can measure relative causal influences on these conclusions. They do this by randomly changing the factor and measuring how it can affect the outcome change. If it can significantly change the outcome, it means it has a huge influence on the decision.
Aggregating influence
The method used by companies can also incorporate different factors that work together. Consider decision systems that grant credit to borrowers who meet at least two criteria: ownership of an automobile, credit rating above 600, or whether the borrower has paid their housing debenture in full.
Say the borrower had a credit rating of 730, didn’t own a housing or auto loan, and was denied credit. They will wonder whether their auto ownership status or housing debenture repayment history is the main reason why they were denied. An analogy can help companies explain how they analyze this type of situation.
Consider courts where decisions are made by majority judges’ votes, where one is a liberal, the other one is a conservative, and the last one is a swing vote, a person who might side with the other party. In two-to-one conservative conclusions, swing judges can greatly influence outcomes compared to liberal judges. Factors in this example are like the three judges mentioned above.
The first judge usually votes in favor of the debenture because most borrowers have high enough credit ratings. These second judge usually votes against the debenture since very few borrowers have ever paid off a housing loan. So the decision will come down to swing judges, who, in this case, reject the credit since the applicant does not own a vehicle.
Companies can do this reasoning accurately by using cooperative game theories, systems of analyzing more specifically how various factors can contribute to most outcomes. Usually, firms will combine their measurements of the relative influences with different values, which is an excellent way to calculate how to assign influences to different factors. Together, these will form Quantitative Input Influence measurements.